The Ultimate Guide to Semantic Segmentation Labeling Tools
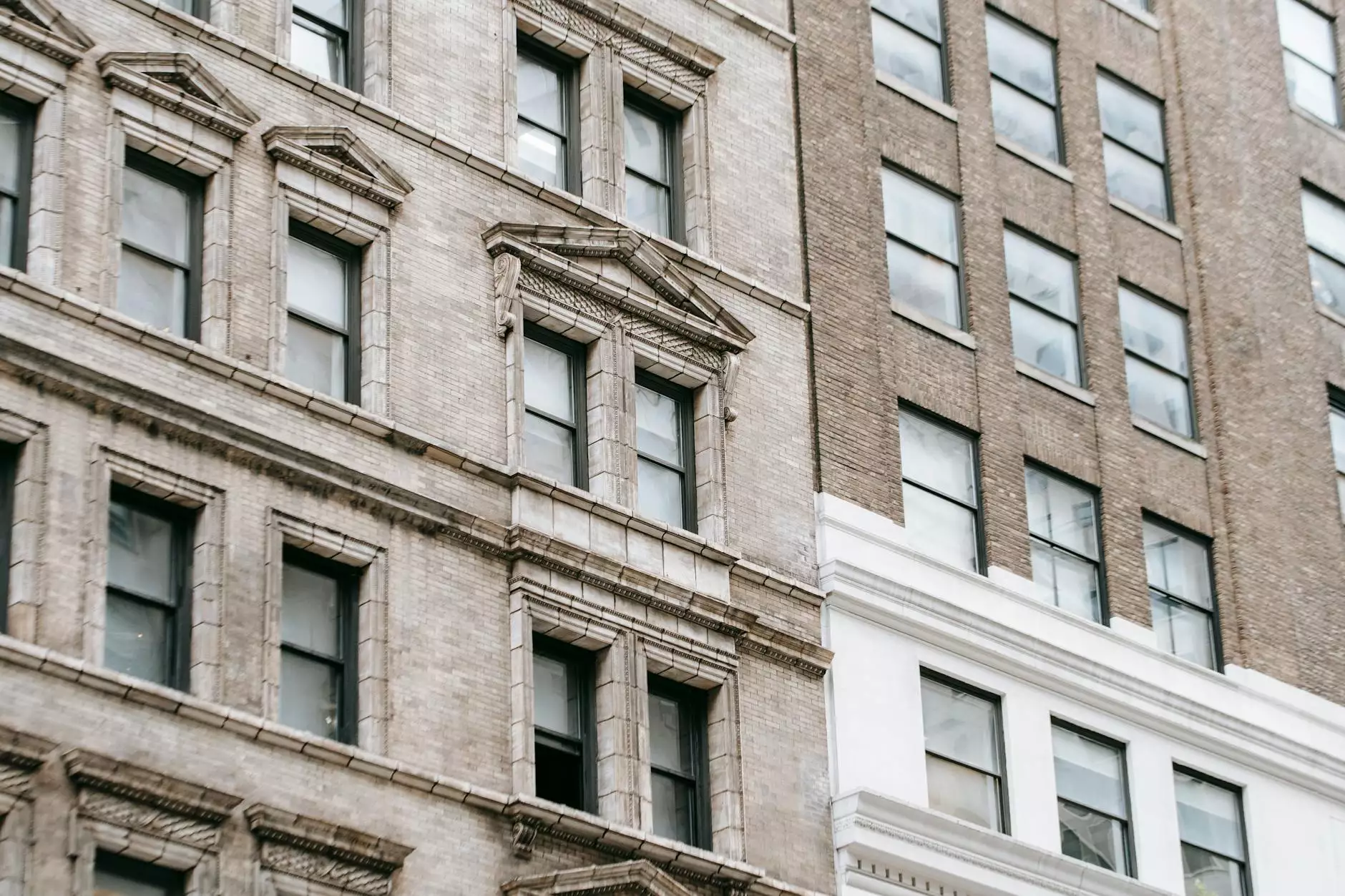
Understanding the importance of data annotation in the realm of artificial intelligence and machine learning is crucial. As AI technologies advance, so does the demand for high-quality annotated datasets. Within this landscape, the role of a semantic segmentation labeling tool emerges as a pivotal component, enabling businesses to effectively prepare their data for AI applications.
What is Semantic Segmentation?
Semantic segmentation is a process in computer vision that involves classifying each pixel in an image into predefined categories. This differentiation provides machines with the ability to understand and interpret images similarly to human perception. Here are the key aspects of semantic segmentation:
- Object Recognition: Identifying and categorizing individual objects within an image.
- Image Understanding: Developing a holistic comprehension of the scene by analyzing pixel-level data.
- Real-time Processing: Facilitating instant analysis and feedback in various applications, including self-driving cars, medical imaging, and robotics.
The Role of Data Annotation Tools in Semantic Segmentation
The process of defining specific areas of interest within images is known as data annotation. The techniques employed in data annotation vary, but semantic segmentation is one of the most sophisticated approaches. This is where semantic segmentation labeling tools come into play. These tools are designed to provide various functionalities that enhance the data annotation process.
Key Features of Semantic Segmentation Labeling Tools
The following features are integral to effective semantic segmentation labeling tools:
- User-friendly Interface: A well-designed user interface allows annotators to easily navigate the tool and efficiently label images.
- Robust Annotation Capabilities: The tool should support a range of labeling types, including point, polygon, and brush tools for precise segmentation.
- Collaboration Features: Enabling teams to collaborate in real-time helps streamline the labeling process and improve overall productivity.
- Integration with Machine Learning Models: The ability to integrate with existing ML frameworks allows for immediate application of labeled data in model training.
- Quality Control Mechanisms: Robust quality assurance features ensure that annotations meet the required standards before being finalized.
Benefits of Using a Semantic Segmentation Labeling Tool
Utilizing a semantic segmentation labeling tool provides a multitude of benefits for businesses engaged in AI and machine learning:
1. Enhanced Data Quality
High-quality annotations are essential for the accuracy of AI models. A powerful labeling tool can significantly improve the precision of pixel-wise annotations, leading to better model predictions.
2. Increased Efficiency
By leveraging advanced features such as auto-segmentation and pre-labeling, annotators can save time, allowing them to focus more on quality rather than manual labeling.
3. Scalability
As the volume of data continues to grow, a robust labeling tool can scale operations, enabling businesses to handle large datasets without compromising on annotation quality.
4. Consistency in Labeling
Automation features help ensure that annotations are consistent across large datasets, reducing the likelihood of human error.
5. Cost-effectiveness
Investing in a quality labeling tool can significantly lower overall annotation costs by streamlining processes and reducing the time needed for manual labor.
Choosing the Right Semantic Segmentation Labeling Tool
When selecting a semantic segmentation labeling tool, consider the following criteria:
- Feature Set: Ensure the tool offers the annotation features you require.
- Ease of Use: A user-friendly interface minimizes the learning curve and increases productivity.
- Support and Documentation: Good customer support and comprehensive documentation are essential for problem-solving.
- Trial or Demo Available: Testing the tool before commitment can help assess its suitability for your specific needs.
- Pricing Model: Evaluate your budget against the pricing structure offered by the tool.
Real-world Applications of Semantic Segmentation
The demand for semantic segmentation is steadily increasing across various industries. Here are some notable applications:
1. Autonomous Vehicles
Autonomous driving systems rely heavily on semantic segmentation to detect and classify objects on the road, including pedestrians, vehicles, and signage.
2. Medical Imaging
In the healthcare sector, semantic segmentation plays a critical role in accurately identifying different tissues and structures in medical scans, enhancing diagnostic processes.
3. Agriculture
In precision agriculture, semantic segmentation helps monitor crop health by analyzing imagery obtained from drones, ensuring better yield predictions and resource allocation.
4. Retail and Surveillance
In retail, understanding customer behavior through image analysis helps businesses design better layouts and marketing strategies. Surveillance uses semantic segmentation for identifying potential threats in public spaces.
The Future of Semantic Segmentation Labeling Tools
The evolution of AI continues to influence the development of semantic segmentation labeling tools. Future advancements may include:
- AI-Assisted Annotation: Integrating advanced AI models to automate much of the segmentation process, reducing the need for manual input.
- Enhanced User Experiences: Developing interfaces that adapt to user preferences and facilitate smoother workflows.
- Cloud-based Solutions: Expanding accessibility and collaboration through cloud technology, allowing teams to work together seamlessly from anywhere.
- Expanded Integration Capabilities: Creating more options for integration with existing workflows, tools, and platforms, enhancing overall productivity.
Conclusion
The advent of semantic segmentation labeling tools represents a significant milestone in the realm of data preparation for machine learning. By ensuring high-quality annotations, these tools lay the foundation for developing more precise and reliable AI applications. Whether you're in the automotive, healthcare, agriculture, or retail industry, adopting a robust labeling tool can result in enhanced data quality, improved efficiency, and ultimately greater success in your AI endeavors.
At Keylabs.ai, we are dedicated to providing cutting-edge data annotation tools and services that empower businesses to thrive in an AI-driven world. Our semantic segmentation labeling tool is designed with precision, efficiency, and user experience in mind, ensuring your data annotation needs are met with the highest standards. Embrace the future of AI with us.