Harnessing Machine Learning for Agriculture: The Role of Datasets
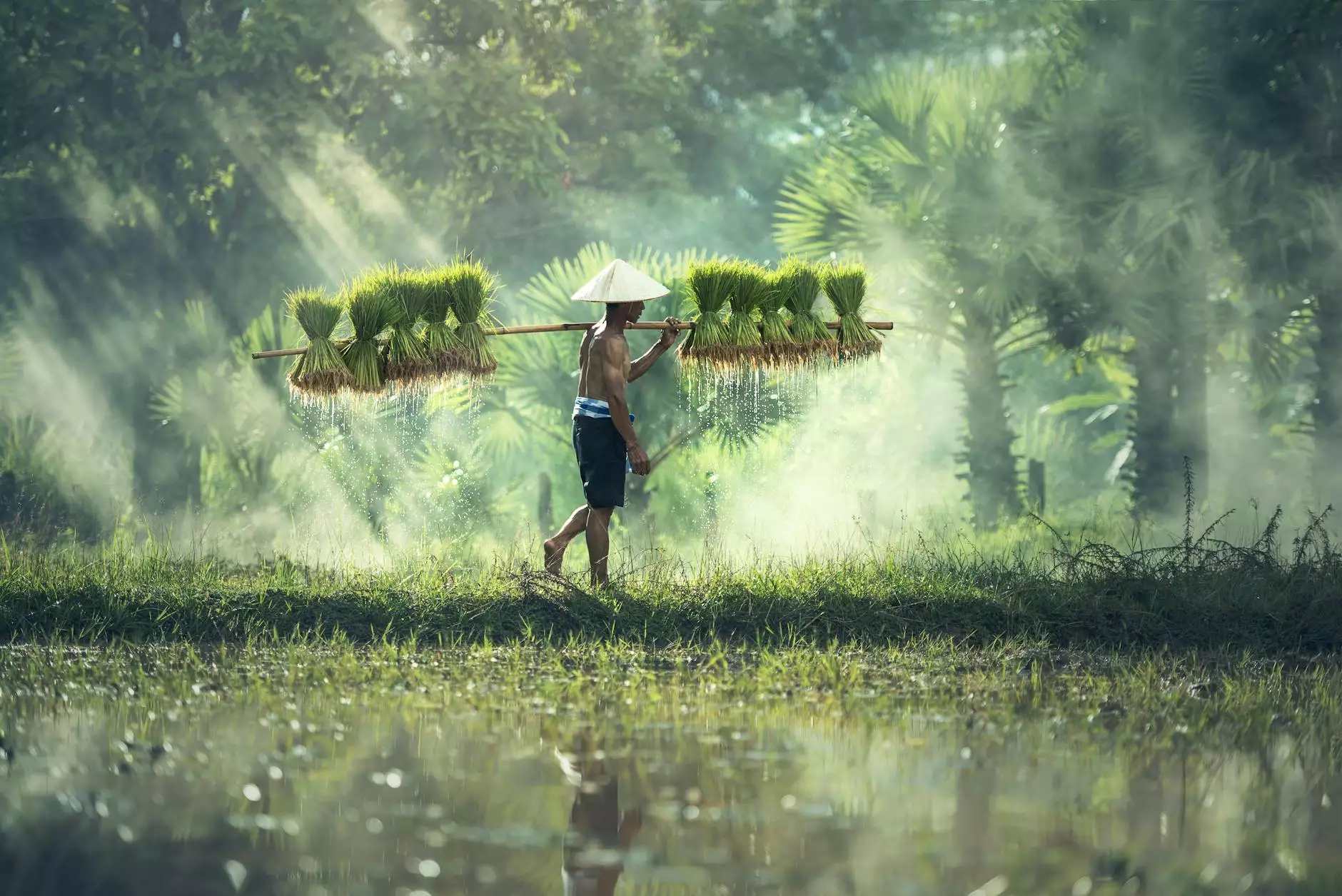
As the world population continues to grow at an unprecedented rate, the need for sustainable and efficient agricultural practices becomes increasingly vital. Agriculture is no longer merely about soil and crops; it's a dynamic field where technology, such as machine learning, plays a significant role. One of the cornerstones to successfully implementing machine learning in agriculture lies in the availability and utilization of robust agriculture datasets for machine learning.
Understanding the Importance of Datasets in Machine Learning
Data is the fuel that powers machine learning algorithms. A high-quality dataset can drastically improve the accuracy and efficiency of machine learning models. In agriculture, datasets are crucial for many applications, including:
- Predictive Analytics: Forecasting crop yields and market prices.
- Pest and Disease Detection: Early identification of crop threats.
- Precision Farming: Enhancing resource allocation through data analysis.
- Crop Classification: Distinguishing between various types of crops for better management.
Types of Agriculture Datasets
When it comes to agriculture datasets for machine learning, there are several different types that can be utilized. Understanding these types can help farmers and agribusinesses make informed decisions on which datasets to leverage for their operations:
1. Climate and Weather Data
Weather conditions significantly affect agricultural productivity. Climate datasets include information on temperature, humidity, rainfall, and sunlight exposure. This data can be used to build models that predict ideal planting times or assess the risk of adverse weather events.
2. Soil Data
Soil health is fundamental to agriculture. Datasets that provide information on soil composition, pH levels, and moisture content can help in managing soil health strategically. This data is essential for developing machine learning models aimed at optimizing crop yield.
3. Crop Yield Data
Historical yield data is one of the most valuable assets for an agribusiness. Analyzing past performances can help predict future outcomes. Machine learning algorithms trained on yield datasets can offer insights into which seeds may perform better under specific conditions.
4. Pest and Disease Incidence Data
Data outlining instances of pest and disease can inform farmers about potential threats to their crops. Analyzing this data with machine learning can yield predictive models that alert farmers before an infestation occurs.
5. Market Data
Market datasets provide pricing information, supply and demand trends, and consumer preferences. This information can be critical for farmers and sellers in making timely decisions about what to plant and when to sell.
The Role of Machine Learning in Agriculture
Machine learning, a subset of artificial intelligence, enables computers to learn from data and improve their performance over time. In the agricultural sector, it is revolutionizing how farmers interact with their land and resources. Here are some practical applications:
1. Yield Prediction
By training models on comprehensive agriculture datasets for machine learning, farmers can accurately predict crop yields. This predictive capability enables better planning for resource allocation, labor, and sales strategies.
2. Precision Agriculture
Precision agriculture employs technologies such as drones and satellite imagery alongside machine learning to enhance productivity. By analyzing various datasets, farmers can apply fertilizers and pesticides only where needed, minimizing waste and environmental impact.
3. Automated Machinery
Machine learning can optimize the operation of farm machinery. For instance, self-driving tractors equipped with sensors can analyze soil and crop conditions in real-time and make autonomous decisions based on the data.
4. Supply Chain Optimization
Machine learning helps optimize several facets of the supply chain from production to distribution. Predictive models allow agribusinesses to manage inventory effectively, reducing costs, and ensuring timely delivery of products to market.
Best Practices for Utilizing Agriculture Datasets
To effectively leverage agriculture datasets for machine learning, it's essential to follow certain best practices:
- Data Quality: Ensure the datasets are accurate, clean, and relevant.
- Data Variety: Utilize multiple types of data for a comprehensive analysis.
- Continuous Updating: Regularly update datasets to reflect new findings and trends.
- Collaborative Efforts: Work with scientists, farmers, and data analysts to share insights and improve data accuracy.
- Ethical Use of Data: Respect privacy and data ownership when using datasets.
Challenges in Using Agriculture Datasets
While the benefits of agriculture datasets for machine learning are immense, there are also challenges that need to be addressed:
1. Data Accessibility
Access to quality datasets can be difficult, especially for smallholder farmers. Often, valuable data is locked behind proprietary systems.
2. Data Integration
Integrating data from various sources—weather stations, soil sensors, and market trends—can be a complex process. This integration is crucial for developing comprehensive machine learning models.
3. Technical Knowledge
The technical expertise required to analyze and interpret complex datasets can be a barrier to entry for many in the agricultural field. Training and education are essential to empower workers to make data-driven decisions.
The Future of Agriculture with Machine Learning
The integration of machine learning into agriculture is only expected to grow. As technology advances and more data becomes available, the potential applications are limitless. Investing in high-quality agriculture datasets for machine learning will become critical for staying competitive in the agricultural industry.
Farmers and agribusinesses that embrace this transformation stand to benefit from increased efficiency, reduced costs, and higher yields. The commitment to continuous learning and adaptation will be necessary to leverage the full potential of machine learning in agriculture.
Conclusion
In conclusion, the agricultural landscape is being reshaped by the incorporation of agriculture datasets for machine learning. By understanding the types of available datasets, the role of machine learning in agriculture, and best practices for utilization, farmers can unlock new levels of productivity and sustainability. The future of agriculture is bright, and embracing data-driven technologies is the key to success.
agriculture dataset for machine learning